loongson/pypi/: compressed-segmentation-2.3.1 metadata and description
Neuroglancer compressed_segmentation codec.
author | Jeremy Maitin-Shepard, Stephen Plaza, and William Silversmith |
author_email | ws9@princeton.edu |
classifiers |
|
description_content_type | text/markdown |
requires_dist |
|
File | Tox results | History |
---|---|---|
compressed_segmentation-2.3.1-cp38-cp38-linux_loongarch64.whl
|
|
Compress Seg 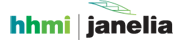
Library for compressing and decompressing image segmentation (adapted from neuroglancer)
import compressed_segmentation as cseg sx, sy, sz = (128,128,128) dtype = np.uint64 order = 'C' labels = np.arange(0, sx*sy*sz, dtype=dtype).reshape((sx,sy,sz), order=order) compressed = cseg.compress(labels, order=order) recovered = cseg.decompress( compressed, (sx,sy,sz) dtype=dtype, order=order ) arr = CompressedSegmentationArray( compressed, shape=(sx,sy,sz), dtype=dtype ) label = arr[54,32,103] # random access to single voxels w/o decompressing uniq_labels = arr.labels() # get all distinct values w/o decompressing binary2 = arr.remap({ 1: 2 }, preserve_missing_labels=False) # remap labels in segmentation w/o decompressing recovered = arr.numpy() # decompress to a numpy array, same as decompress 124213 in arr # test if a value is in the array
cseg compress connectomics.npy cseg decompress connectomics.npy.cseg --volume-size 512,512,512 --bytes 4
NOTE: This repository is the PyPI distribution repo but is based on work done by Jeremy Maitin-Shepard (Google), Stephen Plaza (Janelia Research Campus), and William Silversmith (Princeton) here: https://github.com/janelia-flyem/compressedseg
This library contains routined to decompress and compress segmentation and to manipulate compressed segmentation data defined by the neuroglancer project. compressed_segmentation essentially renumbers large bit width labels to smaller ones in chunks. This provides for large reductions in memory usage and higher compression.
Note that limitations in the compressed_segmentation format restrict the size of the chunk that can be compressed. As this limitation is data dependent, for example a random array with 1024 labels passes testing at 256x256x128, but 256x256x256 often does not.
Features
- Compression and decompression
- Random access to voxels without decompression
- Read out unique values without decompression
- Remap labels without decompression
- Command line interface for numpy files
- (TBD) Interface to relabel and manipulate segmentation from the compressed data
- C++, Python, and Go interface (see original repo for Golang)
C++ Compilation
Compiling as a shared library. Feel free to subsititute e.g. clang for the C++ compiler.
g++ -std=c++11 -O3 -fPIC -shared -I./include src/compress_segmentation.cc src/decompress_segmentation.cc -o compress_segmentation.so
Python Installation
pip
Binary Installation
$ pip install compressed-segmentation $ python >>> import compressed_segmentation as cseg >>> help(cseg)
If there are pre-built binaries available for your architecture this should just work.
pip
Source Installation
If you need to build from source, you will need to have a C++ compiler installed:
$ sudo apt-get install g++ python3-dev $ pip install numpy $ pip install compressed-segmentation $ python >>> import compressed_segmentation as cseg >>> help(cseg)
Direct Installation
Requires a C++ compiler such as g++ or clang.
Works with both Python 2 and 3. Encodes from / decodes to 3D or 4D numpy ndarrays.
$ sudo apt-get install g++ python3-dev $ pip install -r requirements.txt $ python setup.py install $ python >>> import compressed_segmentation as cseg >>> help(cseg)
License
Please see the licenses in this repo.